In this article from the “Datapedia” column, we will explore 4 pairs of terms including Artificial Intelligence and Machine Learning. We will explain clearly when to use them, highlighting their definitions, objectives, use cases, and differences.
“Datapedia” is our monthly column where we illustrate the meaning of terms often misunderstood and confused in the world of Business Intelligence (BI) and Data Visualization.
Start enriching your vocabulary with this reading.
Permissions vs Row Security Level: complementary for information management
Permissions, commonly known as authorizations, refer to the access rights assigned to users or groups of users to perform specific operations on resources or data within a system. These permissions can include operations such as reading, writing, modifying, or deleting data, and are usually assigned at the role or group level.
On the other hand, Row Level Security (RLS) represents an advanced data security technique that limits access to individual records or rows of data within a table based on specific criteria defined by the system administrator.
This approach allows to precisely establish which data each user can view or modify, in relation to their access privileges.
Comparison: Differences and Objectives
The main difference between permissions and RLS lies in the level of granularity of access control. Permissions focus on the actions that users can undertake on various resources or data sets, on the contrary RLS specifically targets the data rows that users are allowed to view or alter within a table.
Permissions aim to manage and control access to resources and data, ensuring that each user has only the necessary authorizations for their activities. In contrast, RLS aims to restrict user access strictly to the data rows that directly pertain to their role or responsibilities.
Advantages, Limitations, and Use Cases
Permissions offer flexible and scalable access management to resources, although they can become complex in the absence of a clear organizational structure. RLS, on the other hand, ensures precise and direct control over data access at the row level, avoiding cumbersome processes and reducing the margin of error.
Permissions are widely used in managing corporate information, allowing, for example, various human resources teams to access specific data in a controlled and secure manner. RLS, however, is prevalent in contexts where it is crucial to limit access to particularly sensitive data. An example is the healthcare sector, where it is essential for doctors to view only the rows related to their own patients’ data.
In summary, while permissions focus on controlling access to objects and data, RLS provides more granular and targeted data access control at the row level. Both are essential tools for ensuring data security and integrity within a system, offering complementary approaches to managing and controlling access.
Wireframe vs Mockup: What to Present to the Client
The wireframe is the skeleton of the user interface, a basic and schematic visual representation made with lines, geometric shapes, and text blocks. Its function is to prioritize the layout and organization of key elements, downplaying the details of graphic design and interactions. Essential in the early stages of design, the wireframe is a tool to outline the basic structure of the interface, promoting a quick and shared evaluation of ideas among development and design teams.
Unlike the wireframe, the mockup is a more elaborate and detailed representation of the user interface. It includes colors, typography, images, and other stylistic elements to provide a more accurate and almost final visualization of the interface. This allows for the evaluation of the product’s usability, offering stakeholders a clear perspective of the anticipated design and eliciting precise feedback on visual and functional aspects.
Comparison: Differences and Objectives
The main difference between a mockup and a wireframe lies in the level of detail and realism of the representation. While the wireframe focuses on the structure and organization of the interface, the mockup provides a more complete and detailed view of the product’s final appearance.
The wireframe trace the structure and organization of the user interface, facilitating the exchange of ideas and communication between the design team, developers, and the client. On the other hand, the mockup aims to offer a realistic and in-depth view of the interface, highlighting aesthetics and interactive functionalities, which is crucial for assessing the usability and visual appeal of the product during development.
Advantages, Limitations, and Use Cases
The wireframe stands out for its ability to provide a clear and concise representation of the user interface structure, being simple and quick to develop. However, it may be less effective in conveying more complex design ideas and concepts. On the other hand, the mockup offers a more realistic and detailed visualization, providing a closer representation of the final product, but its creation and updating require more time and resources.
In practical application, the wireframe is essential in the initial design phases to establish the structure and organization of the interface, often immediately after the initial conceptual discussions. The mockup, however, is crucial in the advanced stages, when evaluating the aesthetics and interactivity of the interface. Both tools, while serving different purposes, complement each other: the wireframe helps to quickly reach a consensus on the structure, while the mockup allows for refining and detailing the design further.
To sum up, while the wireframe focuses on the structure and organization of the user interface, the mockup provides a more detailed and realistic view of the interface’s appearance and interactions. Both are essential tools in the digital product design and development process, offering complementary approaches for communicating and evaluating design with clients.
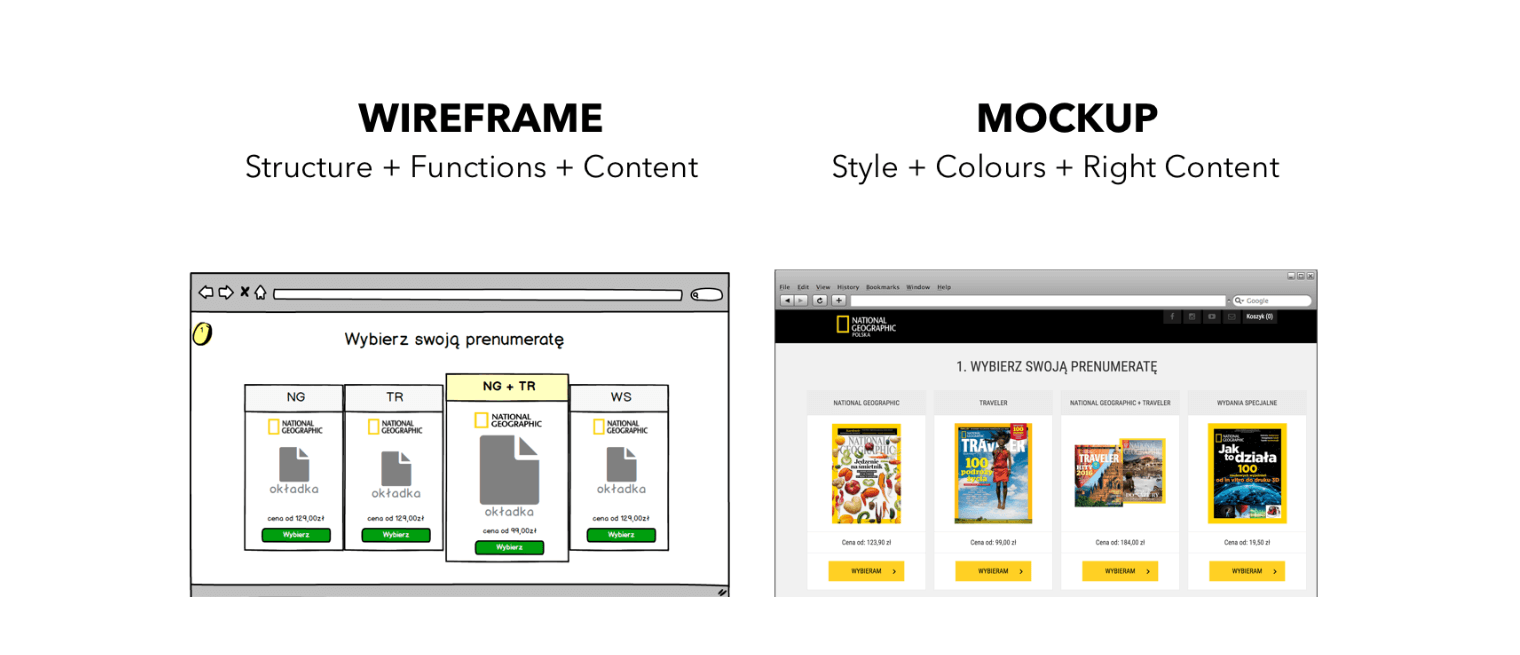
Artificial Intelligence vs Machine Learning: Are They the Same Thing?
Artificial Intelligence (AI) is a field of computer science dedicated to creating systems capable of mimicking human intelligence. These systems are designed to perform tasks such as natural language recognition, visual perception, reasoning, and decision-making.
Within AI, Machine Learning (ML) is a subcategory focused on the ability of machines to learn autonomously from data. This allows systems to improve their performance over time without being explicitly programmed, using algorithms and statistical models.
Comparison: Differences and Objectives
The fundamental distinction between Artificial Intelligence and Machine Learning lies in the fact that AI is a broader field that encompasses ML as one of its areas. While AI deals with creating systems capable of performing intelligent tasks, ML is a specific technique used within AI to train models to learn from data.
The goal of AI is to create systems capable of simulating human activities to automate and enhance the efficiency of processes that can become more precise and consistent. However, machines may struggle with understanding social, cultural, or emotional contexts. On the other hand, Machine Learning aims to develop systems that can autonomously learn from data sets to perform tasks such as prediction, classification, clustering, and optimization. This automatic learning process enables the generation of predictions or decisions based on recognized patterns, although it requires large amounts of quality data and may face challenges such as high costs, difficulty in data acquisition, and the risk of incorporating existing biases in the data, which could lead to unfair or inaccurate decisions.
Use Cases
In terms of use cases, Artificial Intelligence is applied in virtual assistants and chatbots, autonomous driving, and recommendation systems. Meanwhile, Machine Learning is used, for example, in market demand forecasting or predictive medical diagnoses.
In short, while AI represents a broader goal, ML is a specific technique used within AI to train models to learn from data.
Deep Learning vs Internet of Things (IoT): What Are the Differences?
Deep Learning is an advanced Machine Learning technique that relies on deep artificial neural networks for processing complex data. This technology is capable of learning from large amounts of unstructured data, such as images, audio, or text, and extracting high-level models for tasks like pattern recognition, classification, or prediction.
The Internet of Things (IoT), on the other hand, refers to an ecosystem of physical devices, sensors, and other network-connected objects capable of collecting, transmitting, and exchanging data with each other and external systems.
Comparison: Differences and Objectives
The key difference between Deep Learning and IoT lies in their scope of application: Deep Learning is a data analysis technique, while IoT is a physical and conceptual infrastructure enabling the connection and data exchange among smart devices.
While Deep Learning focuses on extracting complex patterns from unstructured data and automating complex tasks such as image recognition or speech recognition, IoT aims to create and implement intelligent solutions to enhance daily life and operational efficiency through the collection and analysis of data from connected devices.
Advantages, Limitations, and Use Cases
Deep Learning provides the ability to process complex data and extract meaningful patterns but requires large amounts of training data and computational resources. In contrast, IoT offers high interoperability and connectivity among devices but may face challenges related to data security and privacy.
Deep Learning is crucial for image and video analysis or voice recognition systems. IoT, on the other hand, is used to monitor and control smart home devices, optimize industrial processes, and improve energy resource management.
In conclusion, while Deep Learning is an advanced data analysis technique, IoT represents a physical and conceptual infrastructure that enables the connection and data exchange among smart devices.
Learn to Design a Dashboard with Figma
If you want to learn the best practices of UX and UI to create effective reports, enroll in our course “Figma for Dashboard Design”.
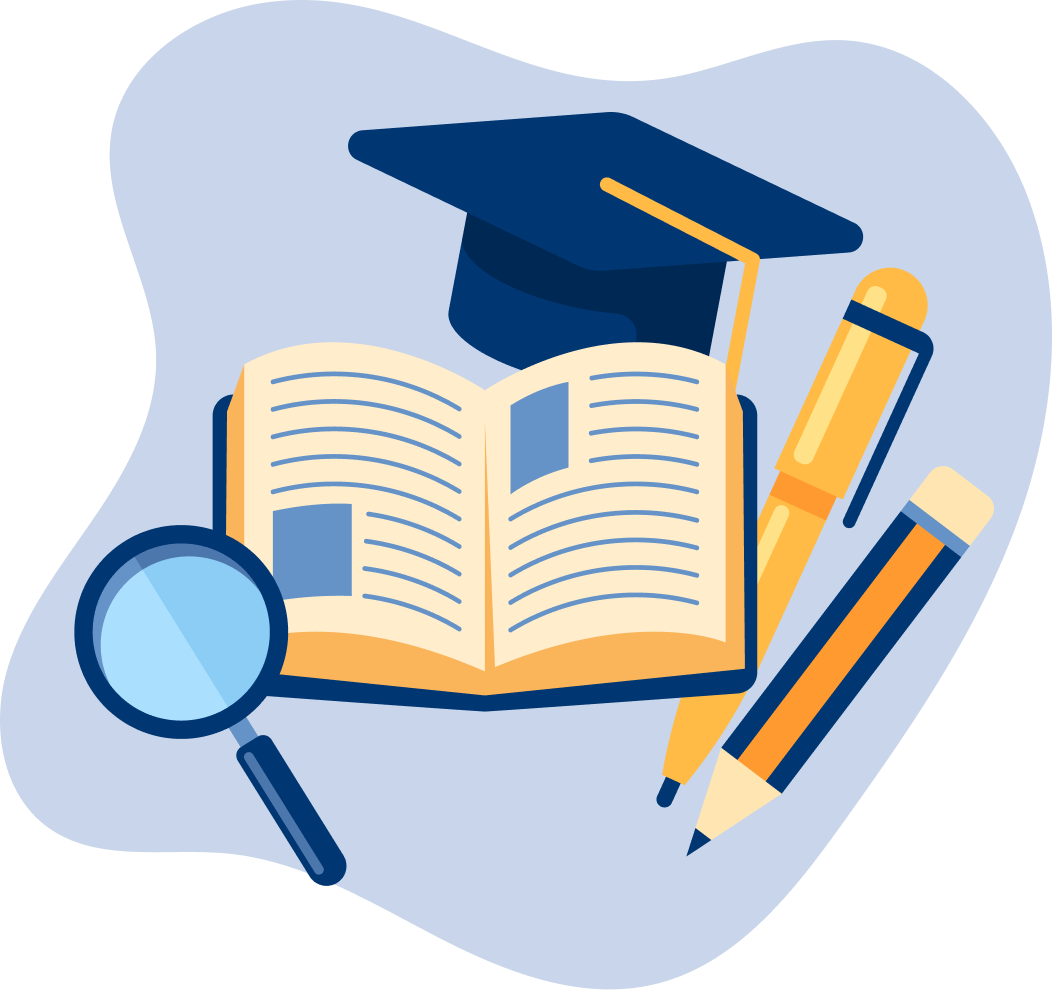
Visualitics Team
This article was written and edited by one of our consultants.
Share now on your social channels or via email: